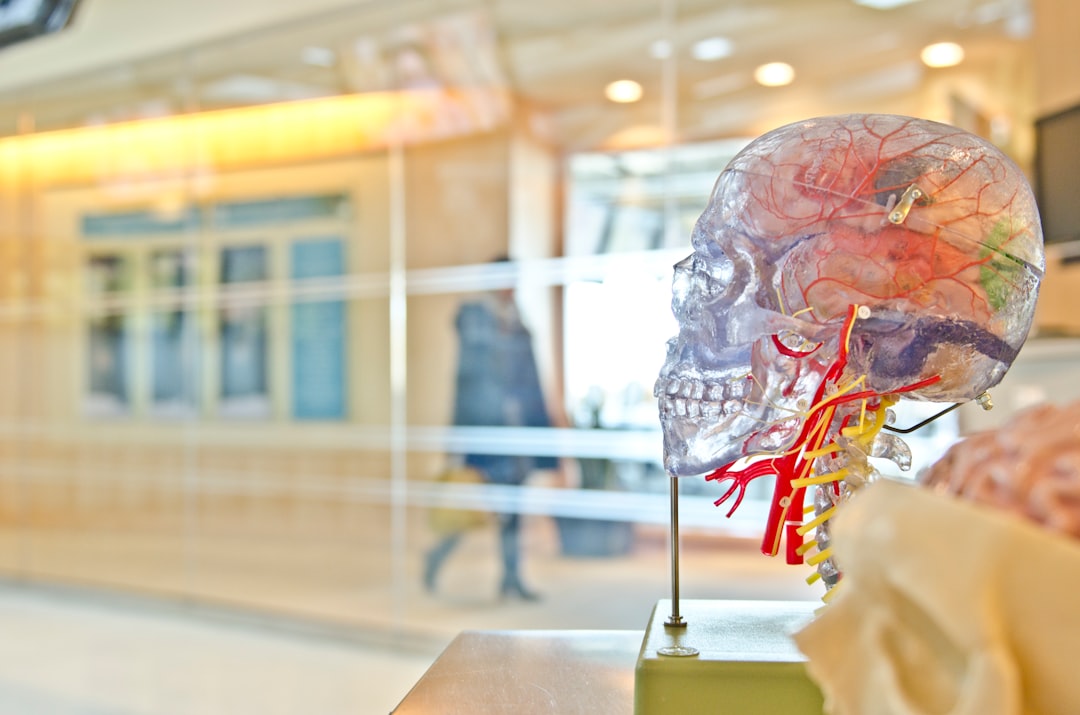
Revolutionizing Cancer Detection with AI Technology
The advent of artificial intelligence (AI) technology has ushered in a new era in the field of medicine, particularly in cancer detection. Cancer remains one of the leading causes of mortality worldwide, with millions diagnosed each year. Traditional methods of cancer detection, such as imaging techniques and biopsies, while effective, often come with limitations in terms of accuracy, speed, and accessibility.
AI technology, particularly through machine learning algorithms, has emerged as a powerful tool that can enhance the diagnostic process, enabling earlier detection and potentially improving patient outcomes. AI’s ability to analyze vast amounts of data quickly and accurately positions it as a game-changer in oncology. By leveraging deep learning techniques, AI systems can identify patterns and anomalies in medical images that may be imperceptible to the human eye.
This capability not only aids radiologists in making more informed decisions but also streamlines the workflow in clinical settings. As healthcare systems continue to grapple with the challenges of rising patient numbers and limited resources, the integration of AI into cancer detection processes offers a promising solution to enhance efficiency and effectiveness.
Key Takeaways
- AI technology has revolutionized cancer detection by using machine learning algorithms to analyze medical images and data.
- Machine learning plays a crucial role in cancer detection by identifying patterns and anomalies in medical images and patient data.
- The advantages of using AI in cancer detection include increased accuracy, early detection, and personalized treatment options.
- Challenges and limitations of AI technology in cancer detection include the need for large and diverse datasets, potential biases, and regulatory hurdles.
- Case studies have shown successful implementation of AI in cancer detection, leading to improved patient outcomes and reduced healthcare costs.
The Role of Machine Learning in Cancer Detection
Machine learning, a subset of AI, plays a pivotal role in revolutionizing cancer detection methodologies. By utilizing algorithms that learn from data, machine learning models can improve their accuracy over time as they are exposed to more cases. In the context of cancer detection, these models can be trained on extensive datasets comprising medical images, pathology reports, and patient histories.
For instance, convolutional neural networks (CNNs) have been particularly successful in analyzing radiological images such as mammograms and CT scans, where they can detect subtle signs of malignancy that may be overlooked by human observers. One notable application of machine learning in cancer detection is in the analysis of histopathological images. Pathologists traditionally examine tissue samples under a microscope to identify cancerous cells, a process that can be time-consuming and subject to human error.
Machine learning algorithms can automate this process by classifying cells based on their morphological features. For example, researchers have developed models that can differentiate between benign and malignant tumors with high accuracy, significantly reducing the time required for diagnosis and allowing pathologists to focus on more complex cases.
Advantages of Using AI in Cancer Detection
The integration of AI technology into cancer detection offers numerous advantages that can significantly enhance patient care. One of the most compelling benefits is the potential for increased accuracy in diagnosis. Studies have shown that AI algorithms can match or even surpass human experts in detecting certain types of cancers.
For instance, a study published in the journal Nature demonstrated that an AI system could detect breast cancer in mammograms with an accuracy rate higher than that of radiologists. This increased precision can lead to earlier interventions, which are crucial for improving survival rates. Another significant advantage is the efficiency that AI brings to the diagnostic process.
Traditional methods often involve multiple steps and consultations before arriving at a diagnosis, which can delay treatment. AI systems can analyze data rapidly, providing immediate feedback to healthcare providers. This speed not only enhances patient experience but also optimizes resource allocation within healthcare facilities.
For example, AI-driven triage systems can prioritize patients based on their risk levels, ensuring that those who need urgent care receive it without unnecessary delays.
Challenges and Limitations of AI Technology in Cancer Detection
Challenges and Limitations | AI Technology in Cancer Detection |
---|---|
Accuracy | AI algorithms may not always be accurate in detecting cancer cells, leading to false positives or false negatives. |
Data Quality | The quality and quantity of data used to train AI models can impact their effectiveness in cancer detection. |
Interpretability | AI models may lack transparency in their decision-making process, making it difficult to understand how they arrive at their conclusions. |
Regulatory Approval | AI technologies for cancer detection may face challenges in obtaining regulatory approval for clinical use. |
Integration with Healthcare Systems | Integrating AI technology into existing healthcare systems and workflows can be complex and challenging. |
Despite its promising potential, the implementation of AI technology in cancer detection is not without challenges and limitations. One major concern is the quality and representativeness of the data used to train machine learning models. If the training datasets are biased or lack diversity, the resulting algorithms may not perform well across different populations or types of cancers.
This issue raises questions about the generalizability of AI systems and their ability to provide equitable care for all patients. Moreover, there are technical challenges associated with integrating AI into existing healthcare workflows. Many healthcare providers may lack the necessary infrastructure or expertise to implement AI solutions effectively.
Additionally, concerns about data privacy and security are paramount, as sensitive patient information must be protected from breaches while still being utilized for training algorithms. The regulatory landscape surrounding AI in healthcare is also evolving, with agencies like the FDA working to establish guidelines for the approval and monitoring of AI-based diagnostic tools.
Case Studies: Successful Implementation of AI in Cancer Detection
Several case studies illustrate the successful implementation of AI technology in cancer detection, showcasing its transformative potential. One prominent example is Google’s DeepMind Health project, which developed an AI system capable of detecting breast cancer from mammograms with remarkable accuracy. In a clinical trial involving thousands of mammograms from women in the UK and the US, the AI system demonstrated a reduction in false positives and false negatives compared to human radiologists.
This success not only highlights the efficacy of AI but also underscores its potential to alleviate some of the burdens faced by radiologists. Another noteworthy case is PathAI, a company focused on improving pathology through machine learning. PathAI’s algorithms assist pathologists by providing second opinions on diagnoses made from histopathological slides.
In one study, PathAI’s system achieved an accuracy rate exceeding 90% in identifying breast cancer from tissue samples. By augmenting pathologists’ capabilities rather than replacing them, PathAI exemplifies how AI can enhance diagnostic accuracy while preserving the essential human element in patient care.
Future Prospects and Developments in AI Technology for Cancer Detection
Integrating Multi-Modal Data Sources
One area of focus is the integration of multi-modal data sources into AI models. By combining imaging data with genomic information and electronic health records, researchers aim to create more comprehensive models that can provide insights into not only whether cancer is present but also its likely progression and response to treatment.
Advancements in Natural Language Processing
Additionally, advancements in natural language processing (NLP) are expected to enhance AI’s capabilities in analyzing unstructured data such as clinical notes and research articles. This could lead to more informed decision-making by synthesizing vast amounts of information into actionable insights for oncologists.
Towards Personalized Cancer Detection
As machine learning techniques continue to evolve, we may see the emergence of personalized cancer detection systems that tailor screening protocols based on individual risk factors and genetic predispositions.
Ethical Considerations in Using AI for Cancer Detection
The deployment of AI technology in cancer detection raises several ethical considerations that must be addressed to ensure responsible use. One primary concern is the potential for algorithmic bias, which could lead to disparities in care if certain demographic groups are underrepresented in training datasets. It is crucial for developers to prioritize diversity in data collection and validation processes to mitigate these risks.
Furthermore, transparency in AI decision-making processes is essential for building trust among healthcare providers and patients alike. Clinicians must understand how AI systems arrive at their conclusions to effectively interpret results and communicate them to patients. This transparency is vital not only for clinical practice but also for regulatory compliance and accountability.
The Impact of AI Technology on Revolutionizing Cancer Detection
The impact of AI technology on cancer detection is profound and multifaceted, offering unprecedented opportunities for improving diagnostic accuracy and efficiency. As machine learning algorithms continue to evolve and integrate into clinical workflows, they hold the potential to transform how healthcare providers approach cancer diagnosis and treatment planning. While challenges remain regarding data quality, ethical considerations, and integration into existing systems, ongoing research and collaboration among stakeholders will be crucial in overcoming these hurdles.
As we look toward the future, it is clear that AI will play an increasingly central role in oncology, paving the way for more personalized and effective approaches to cancer care. The journey toward fully realizing this potential will require a concerted effort from researchers, clinicians, policymakers, and technology developers to ensure that AI serves as a tool for enhancing human expertise rather than replacing it. The promise of AI technology lies not only in its ability to detect cancer more accurately but also in its capacity to empower healthcare professionals and improve patient outcomes on a global scale.
FAQs
What is an AI-based early cancer detection system?
An AI-based early cancer detection system is a technology that uses artificial intelligence algorithms to analyze medical imaging data, such as mammograms, MRIs, or CT scans, to identify potential signs of cancer at an early stage.
How does an AI-based early cancer detection system work?
AI-based early cancer detection systems use machine learning algorithms to analyze large amounts of medical imaging data and identify patterns or abnormalities that may indicate the presence of cancer. These systems can then provide diagnostic assistance to healthcare professionals in detecting cancer at an early stage.
What are the benefits of AI-based early cancer detection systems?
AI-based early cancer detection systems can help improve the accuracy and efficiency of cancer diagnosis, leading to earlier detection and treatment. This can potentially improve patient outcomes and reduce healthcare costs associated with late-stage cancer treatment.
Are AI-based early cancer detection systems widely used in clinical practice?
While AI-based early cancer detection systems are being developed and tested in clinical settings, they are not yet widely used in routine clinical practice. Research and development in this field are ongoing, and regulatory approval is required before these systems can be implemented on a larger scale.
What are the challenges associated with AI-based early cancer detection systems?
Challenges associated with AI-based early cancer detection systems include the need for large and diverse datasets for training the algorithms, ensuring the accuracy and reliability of the system, and addressing ethical and regulatory considerations related to the use of AI in healthcare. Ongoing research and collaboration between healthcare professionals and technology experts are essential to address these challenges.