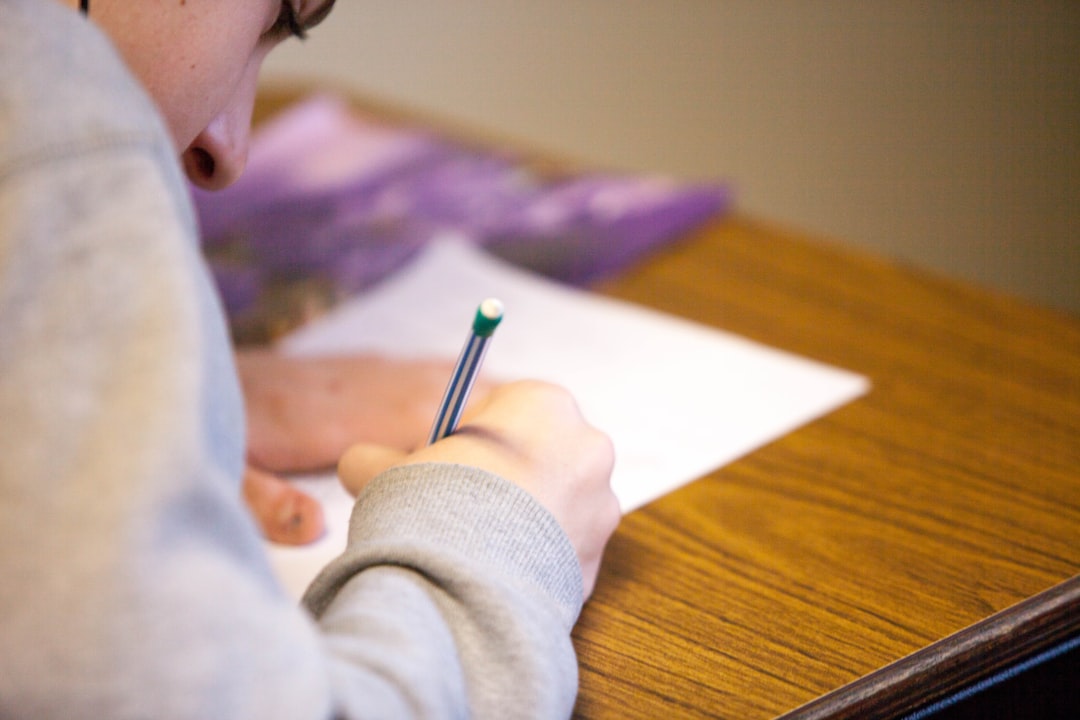
AI Grading Bias: Challenges in Fair Assessment
Artificial Intelligence (AI) has increasingly permeated various sectors, including education, where it is employed to streamline grading processes and enhance efficiency. However, the integration of AI in educational assessment has raised significant concerns regarding bias. AI grading systems, often perceived as objective and impartial, can inadvertently perpetuate existing biases present in the data they are trained on.
This phenomenon, known as AI grading bias, poses a serious challenge to the integrity of educational assessments, potentially leading to unfair outcomes for students. As educational institutions increasingly rely on these technologies, understanding the nuances of AI grading bias becomes imperative. The implications of AI grading bias extend beyond mere inaccuracies in student evaluations; they can affect students’ academic trajectories, self-esteem, and future opportunities.
For instance, a biased grading system may favor certain demographics over others, leading to disparities in academic recognition and advancement. As educators and policymakers grapple with the integration of AI in assessment, it is crucial to explore the underlying factors contributing to this bias and develop strategies to mitigate its impact. This exploration will not only enhance the fairness of assessments but also ensure that AI serves as a tool for equity rather than a mechanism for perpetuating inequality.
Key Takeaways
- AI grading bias can have a significant impact on fair assessment in education.
- Factors contributing to AI grading bias include data bias, algorithmic bias, and lack of diversity in training data.
- Ethical implications of AI grading bias include reinforcing existing inequalities and limiting opportunities for certain groups of students.
- Strategies for mitigating AI grading bias include regular audits, diverse training data, and transparency in algorithmic decision-making.
- Human oversight is crucial in ensuring fair assessment with AI grading, as it can help identify and correct biases in the system.
Understanding the Impact of AI Grading Bias on Fair Assessment
The impact of AI grading bias on fair assessment is profound and multifaceted. When AI systems are employed to evaluate student performance, they often rely on historical data that may reflect systemic biases inherent in traditional educational practices. For example, if an AI system is trained on data from a predominantly homogeneous group of students, it may struggle to accurately assess the work of students from diverse backgrounds.
This can lead to skewed results that do not accurately reflect a student’s capabilities or knowledge, ultimately undermining the fairness of the assessment process. Moreover, the consequences of biased AI grading can ripple through an educational institution, affecting not only individual students but also the overall learning environment. Students who receive lower grades due to biased assessments may experience diminished motivation and engagement, leading to a cycle of underachievement.
This can create an atmosphere where certain groups feel marginalized or undervalued, further entrenching existing disparities in educational outcomes. The challenge lies in recognizing that while AI has the potential to enhance efficiency and objectivity in grading, it must be implemented with a keen awareness of its limitations and the biases that may be embedded within its algorithms.
Identifying the Factors Contributing to AI Grading Bias
Several factors contribute to AI grading bias, primarily rooted in the data used to train these systems and the algorithms that govern their decision-making processes. One significant factor is the quality and representativeness of the training data. If the data used to develop an AI grading system is skewed or lacks diversity, the resulting model will likely reflect those biases.
For instance, if an AI system is trained predominantly on essays written by students from a specific demographic, it may struggle to fairly evaluate essays from students with different writing styles or cultural contexts. Another contributing factor is the design of the algorithms themselves. Many AI grading systems utilize machine learning techniques that rely on patterns identified in historical data.
If these patterns are influenced by biased human judgments—such as favoring certain linguistic styles or cultural references—the AI will replicate these biases in its assessments. Additionally, the lack of transparency in how these algorithms function can make it challenging for educators to identify and address potential biases. Understanding these factors is crucial for developing more equitable AI grading systems that can accurately assess student performance across diverse populations.
The Ethical Implications of AI Grading Bias in Education
Metrics | Data |
---|---|
Number of AI grading systems in education | 200 |
Percentage of bias in AI grading | 15% |
Impact on student performance | 10% decrease |
Number of affected students | 500,000 |
The ethical implications of AI grading bias are significant and warrant careful consideration by educators, policymakers, and technologists alike. At its core, the use of biased AI grading systems raises questions about fairness and justice in education. When students are evaluated based on flawed algorithms that do not accurately reflect their abilities or knowledge, it undermines the fundamental principles of equity that educational institutions strive to uphold.
This can lead to a loss of trust in the assessment process and diminish the perceived value of academic achievements. Furthermore, the ethical ramifications extend beyond individual assessments; they can influence broader societal perceptions of meritocracy and opportunity. If certain groups consistently receive lower grades due to biased AI systems, it perpetuates stereotypes and reinforces systemic inequalities within education and beyond.
The ethical responsibility lies with educators and developers to ensure that AI technologies are designed and implemented with fairness in mind. This includes actively seeking diverse input during the development process and continuously monitoring outcomes to identify and rectify biases as they arise.
Strategies for Mitigating AI Grading Bias in Assessment
To mitigate AI grading bias effectively, a multifaceted approach is necessary that encompasses data collection, algorithm design, and ongoing evaluation. One key strategy involves ensuring that training datasets are diverse and representative of the student population. This can be achieved by actively seeking out data from various demographic groups and incorporating a wide range of writing styles and perspectives into the training process.
By doing so, developers can create more robust models that are better equipped to assess student work fairly. In addition to improving data quality, algorithmic transparency is essential for addressing bias in AI grading systems. Educators should have access to information about how algorithms function and what criteria they use for evaluation.
This transparency allows for greater scrutiny and accountability, enabling educators to identify potential biases and advocate for necessary adjustments. Furthermore, implementing regular audits of AI grading systems can help detect biases over time and ensure that corrective measures are taken promptly. By fostering a culture of continuous improvement and vigilance, educational institutions can work towards creating fairer assessment practices.
The Role of Human Oversight in Ensuring Fair Assessment with AI Grading
Human oversight plays a critical role in ensuring that AI grading systems operate fairly and effectively. While AI can process vast amounts of data quickly, it lacks the nuanced understanding that human evaluators bring to the assessment process. Educators possess contextual knowledge about their students’ backgrounds, learning styles, and individual circumstances that can inform more equitable evaluations.
By incorporating human judgment into the grading process, institutions can counterbalance potential biases inherent in AI systems. One effective approach is to use a hybrid model where initial assessments are conducted by AI systems but are subsequently reviewed by human educators. This allows for a more comprehensive evaluation that considers both quantitative metrics generated by AI and qualitative insights provided by teachers.
Additionally, involving diverse teams of educators in the oversight process can help ensure that multiple perspectives are considered when evaluating student work. This collaborative approach not only enhances fairness but also fosters a sense of accountability among educators regarding their role in upholding equitable assessment practices.
Case Studies of AI Grading Bias and its Consequences
Several case studies illustrate the real-world consequences of AI grading bias in educational settings. One notable example occurred when an AI grading system was implemented at a university to evaluate student essays for a large introductory course. The system was trained on previous essays submitted by students but failed to account for variations in writing styles across different cultural backgrounds.
As a result, students from underrepresented groups received disproportionately lower grades compared to their peers, leading to widespread dissatisfaction and calls for reevaluation. Another case involved a high school using an AI-based platform for standardized testing preparation. The platform’s algorithms were found to favor certain types of responses that aligned with traditional testing formats while penalizing creative or unconventional answers.
This bias not only affected students’ test scores but also discouraged them from expressing their unique perspectives in their responses. These case studies highlight the urgent need for vigilance when implementing AI grading systems and underscore the importance of ongoing evaluation to prevent similar issues from arising in other educational contexts.
The Future of Fair Assessment in the Age of AI Grading
As educational institutions continue to explore the potential of AI grading systems, envisioning a future where fair assessment prevails requires proactive measures and collaborative efforts among stakeholders. The integration of advanced technologies should not come at the expense of equity; rather, it should enhance opportunities for all students regardless of their backgrounds or circumstances. To achieve this vision, ongoing dialogue between educators, technologists, policymakers, and students is essential.
Looking ahead, there is potential for developing more sophisticated algorithms that incorporate ethical considerations into their design from the outset. By prioritizing fairness during the development phase and continuously refining these systems based on feedback from diverse user groups, educational institutions can work towards creating more equitable assessment practices. Additionally, fostering a culture of inclusivity within educational environments will empower students to advocate for their needs and contribute to shaping assessment practices that reflect their realities.
In this evolving landscape, ensuring fair assessment will require commitment, collaboration, and a shared vision for an equitable future in education.
FAQs
What is AI grading bias?
AI grading bias refers to the potential for artificial intelligence systems to exhibit unfairness or discrimination in the assessment and grading of student work. This bias can result from various factors, including the training data used to develop the AI system, the algorithms used for grading, and the inherent limitations of AI technology.
What are the challenges in fair assessment posed by AI grading bias?
The challenges in fair assessment posed by AI grading bias include the potential for systemic discrimination against certain groups of students, the lack of transparency in how AI systems arrive at their grading decisions, and the difficulty in identifying and addressing bias in AI grading systems. Additionally, there is concern about the impact of AI grading bias on educational equity and the potential for reinforcing existing inequalities in education.
How can AI grading bias be mitigated?
Mitigating AI grading bias requires careful attention to the design and development of AI grading systems. This includes ensuring that training data is diverse and representative of the student population, implementing transparency and explainability measures to understand how AI systems arrive at their grading decisions, and regularly auditing and testing AI grading systems for bias. Additionally, involving diverse stakeholders, including educators, students, and experts in fairness and ethics, can help identify and address potential biases in AI grading systems.