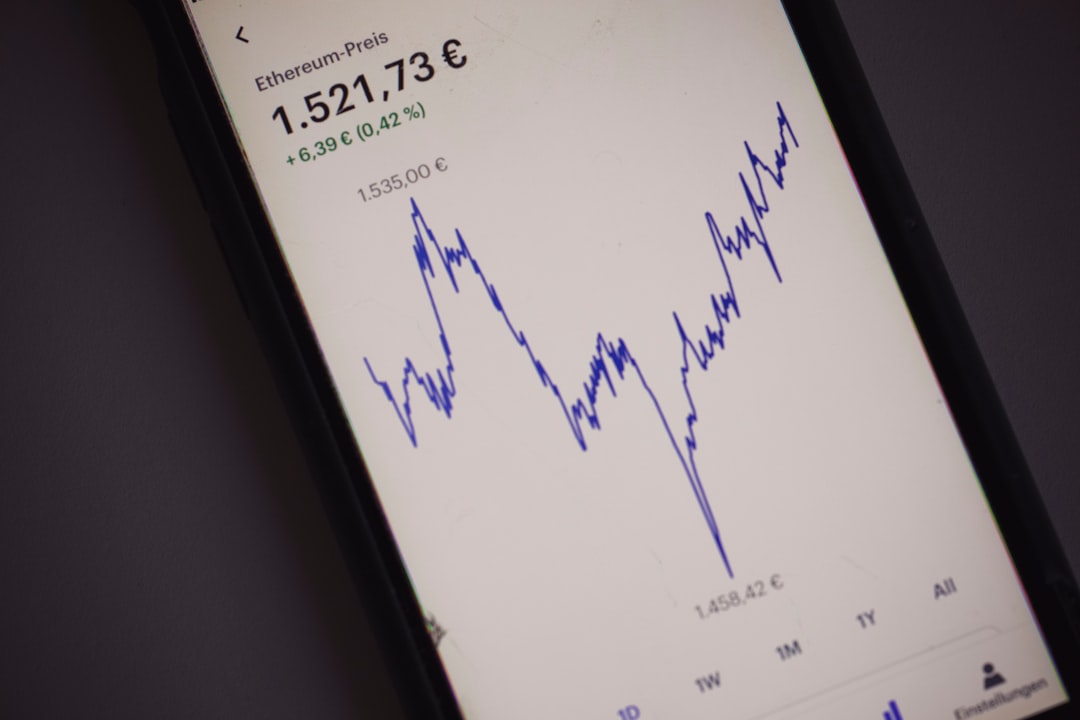
Enhancing Stock Trading with AI Risk Prediction Models
The financial markets have long been characterized by their complexity and volatility, presenting both opportunities and risks for investors. In recent years, the advent of artificial intelligence (AI) has revolutionized the way traders approach stock trading, particularly in the realm of risk prediction. AI risk prediction models leverage vast amounts of data and sophisticated algorithms to forecast potential market movements and identify risks associated with various investment strategies.
These models are designed to analyze historical data, recognize patterns, and make predictions that can significantly enhance trading decisions. As the stock market continues to evolve, the integration of AI into trading strategies has become increasingly essential. Traditional methods of risk assessment often rely on historical performance and fundamental analysis, which can be limited in their predictive power.
In contrast, AI risk prediction models utilize machine learning techniques to adapt and learn from new data, allowing them to provide more accurate and timely insights. This shift towards AI-driven approaches not only enhances the efficiency of trading operations but also empowers traders to make informed decisions in an environment that is often unpredictable.
Key Takeaways
- AI risk prediction models use advanced algorithms to analyze stock market data and predict potential risks in trading.
- AI plays a crucial role in stock trading by providing real-time analysis, pattern recognition, and decision-making support.
- The benefits of using AI risk prediction models in stock trading include improved accuracy, reduced human error, and faster decision-making.
- AI risk prediction models can improve decision-making in stock trading by providing valuable insights, identifying potential risks, and optimizing trading strategies.
- Challenges and limitations of AI risk prediction models in stock trading include data quality issues, algorithmic biases, and the need for human oversight.
- Implementing AI risk prediction models in stock trading strategies requires thorough testing, integration with existing systems, and continuous monitoring.
- Case studies of successful implementation of AI risk prediction models in stock trading demonstrate improved trading performance and risk management.
- The future of AI risk prediction models in stock trading is promising, with advancements in machine learning, big data analytics, and automation shaping the industry.
Understanding the Role of AI in Stock Trading
AI plays a multifaceted role in stock trading, encompassing various technologies such as machine learning, natural language processing, and neural networks. At its core, AI enables traders to process and analyze large datasets far beyond human capabilities. For instance, machine learning algorithms can sift through millions of historical stock prices, economic indicators, and news articles to identify correlations and trends that may not be immediately apparent.
This capability allows traders to develop models that can predict future price movements based on a multitude of factors. Natural language processing (NLP) is another critical component of AI in stock trading. By analyzing news articles, social media sentiment, and earnings reports, NLP algorithms can gauge market sentiment and its potential impact on stock prices.
For example, a sudden surge in negative sentiment surrounding a company due to a scandal can be detected by NLP tools, prompting traders to adjust their positions accordingly. The ability to integrate diverse data sources into a cohesive analysis framework is what sets AI apart from traditional trading methods.
The Benefits of Using AI Risk Prediction Models in Stock Trading
The advantages of employing AI risk prediction models in stock trading are manifold. One of the most significant benefits is the enhancement of predictive accuracy. Traditional risk assessment methods often rely on static models that may not account for sudden market shifts or emerging trends.
In contrast, AI models continuously learn from new data, allowing them to adapt their predictions in real-time. This dynamic approach can lead to more accurate forecasts and better risk management. Moreover, AI risk prediction models can process vast amounts of data at unprecedented speeds.
This capability enables traders to react swiftly to market changes, capitalizing on opportunities that may arise within seconds. For instance, during periods of high volatility, such as earnings announcements or geopolitical events, AI models can quickly analyze the implications of new information and provide actionable insights. This speed and efficiency are crucial in a market where timing can significantly impact profitability.
How AI Risk Prediction Models Can Improve Decision Making in Stock Trading
Metrics | Description |
---|---|
Accuracy | The percentage of correct predictions made by the AI risk prediction model. |
Precision | The ratio of true positive predictions to the total positive predictions made by the model. |
Recall | The ratio of true positive predictions to the total actual positive instances in the data. |
F1 Score | The harmonic mean of precision and recall, providing a balance between the two metrics. |
Confusion Matrix | A table that shows the true positive, false positive, true negative, and false negative predictions made by the model. |
AI risk prediction models enhance decision-making processes by providing traders with data-driven insights that inform their strategies. By utilizing these models, traders can evaluate potential risks associated with specific investments more effectively. For example, an AI model might analyze a stock’s historical volatility alongside macroeconomic indicators to assess the likelihood of a price drop following an earnings report.
This information allows traders to make more informed decisions about whether to hold or sell their positions. Furthermore, AI models can assist in portfolio optimization by identifying the best asset allocation strategies based on risk tolerance and market conditions. By simulating various scenarios and analyzing potential outcomes, these models help traders understand the trade-offs between risk and return.
For instance, an investor looking to minimize risk might use an AI model to determine the optimal mix of stocks and bonds that aligns with their investment goals while maintaining a desired level of risk exposure.
Challenges and Limitations of AI Risk Prediction Models in Stock Trading
Despite their numerous advantages, AI risk prediction models are not without challenges and limitations. One significant concern is the quality of data used to train these models. Inaccurate or biased data can lead to flawed predictions, potentially resulting in substantial financial losses.
For instance, if an AI model is trained on historical data that does not accurately reflect current market conditions or investor behavior, its predictions may be misleading. Another challenge lies in the interpretability of AI models. Many advanced machine learning algorithms operate as “black boxes,” making it difficult for traders to understand how decisions are made.
This lack of transparency can be problematic, especially in high-stakes environments like stock trading where understanding the rationale behind predictions is crucial for trust and accountability. Traders may be hesitant to rely on models they cannot fully comprehend, which could limit the adoption of AI technologies in some cases.
Implementing AI Risk Prediction Models in Stock Trading Strategies
The successful implementation of AI risk prediction models requires a strategic approach that encompasses several key steps. First and foremost, organizations must invest in robust data infrastructure capable of collecting and processing large volumes of relevant data. This infrastructure should include not only historical stock prices but also alternative data sources such as social media sentiment, economic indicators, and geopolitical events that could influence market dynamics.
Once the data infrastructure is established, the next step involves selecting appropriate machine learning algorithms tailored to specific trading objectives. Different algorithms may be better suited for various tasks; for example, time series forecasting models might be ideal for predicting stock prices over time, while classification algorithms could be used to identify potential buy or sell signals based on specific criteria. Collaborating with data scientists and financial analysts is essential during this phase to ensure that the chosen models align with the organization’s overall trading strategy.
Case Studies of Successful Implementation of AI Risk Prediction Models in Stock Trading
Several notable case studies illustrate the successful implementation of AI risk prediction models in stock trading. One prominent example is Renaissance Technologies, a quantitative hedge fund known for its use of advanced mathematical models and algorithms to drive trading decisions. Renaissance employs machine learning techniques to analyze vast datasets and identify patterns that inform its trading strategies.
The firm’s Medallion Fund has consistently outperformed the market over the years, largely attributed to its sophisticated use of AI-driven risk prediction models. Another compelling case is that of Goldman Sachs, which has integrated AI into its trading operations through its Marquee platform. This platform utilizes machine learning algorithms to provide clients with real-time insights into market trends and risks associated with specific trades.
By leveraging AI technology, Goldman Sachs has enhanced its ability to manage risk effectively while delivering value-added services to its clients.
The Future of AI Risk Prediction Models in Stock Trading
Looking ahead, the future of AI risk prediction models in stock trading appears promising as technology continues to advance at an unprecedented pace. As machine learning algorithms become more sophisticated and capable of processing increasingly complex datasets, their predictive power is expected to improve significantly. This evolution will likely lead to more accurate forecasts and enhanced risk management strategies across the financial industry.
Moreover, the integration of AI with other emerging technologies such as blockchain could further transform stock trading practices. Blockchain’s decentralized nature offers enhanced transparency and security for transactions, while AI can provide real-time insights into market conditions and risks associated with specific trades. The convergence of these technologies has the potential to create a more efficient and resilient financial ecosystem.
In conclusion, as financial markets become more intricate and interconnected, the role of AI risk prediction models will continue to expand. By harnessing the power of artificial intelligence, traders can navigate the complexities of stock trading with greater confidence and precision, ultimately leading to improved outcomes in an ever-evolving landscape.
FAQs
What are AI risk prediction models in stock trading?
AI risk prediction models in stock trading are algorithms that use artificial intelligence and machine learning techniques to analyze historical stock data, market trends, and other relevant factors to predict the potential risks associated with trading certain stocks.
How do AI risk prediction models work in stock trading?
AI risk prediction models work by analyzing large amounts of historical stock data, market trends, and other relevant factors to identify patterns and correlations that can be used to predict potential risks associated with trading certain stocks. These models use advanced algorithms and machine learning techniques to continuously learn and improve their predictive capabilities.
What are the benefits of using AI risk prediction models in stock trading?
The benefits of using AI risk prediction models in stock trading include the ability to make more informed trading decisions, identify potential risks and opportunities, and improve overall portfolio management. These models can also help traders and investors mitigate potential losses and optimize their investment strategies.
What are the limitations of AI risk prediction models in stock trading?
Some limitations of AI risk prediction models in stock trading include the potential for inaccuracies or errors in predictions, the reliance on historical data which may not always be indicative of future market behavior, and the need for continuous monitoring and adjustment to account for changing market conditions.
How are AI risk prediction models developed and trained for stock trading?
AI risk prediction models for stock trading are developed and trained using historical stock data, market trends, and other relevant factors. These models are trained using machine learning techniques such as supervised learning, unsupervised learning, and reinforcement learning to identify patterns and correlations that can be used to predict potential risks associated with trading certain stocks.
What are some examples of AI risk prediction models used in stock trading?
Some examples of AI risk prediction models used in stock trading include neural networks, decision trees, support vector machines, and Bayesian networks. These models are used by financial institutions, hedge funds, and individual traders to analyze market data and make informed trading decisions.